データを入力します。
## パッケージの読み込み
library(rstan)
library(tidyverse)
## データの準備
X <- c(-27.020,3.570,8.191,9.808,9.603,9.945,10.056)
sc7 <- list(N=NROW(X),X=X)
ソースコード
## data{
## int<lower=0> N;
## real X[N];
## }
##
## parameters{
## real mu;
## real<lower=0> sig[N];
## }
##
## model{
## for(n in 1:N){
## //likelihood
## X[n] ~ normal(mu,sig[n]);
## //prior
## sig[n] ~ inv_gamma(0.0001,0.0001);
## }
## // prior
## mu ~ normal(0,1000);
## }
推定
fit <- sampling(model,sc7,iter=3000,warmup=1000,chains=4,thin=1)
## Warning: There were 1917 divergent transitions after warmup. Increasing adapt_delta above 0.8 may help. See
## http://mc-stan.org/misc/warnings.html#divergent-transitions-after-warmup
## Warning: Examine the pairs() plot to diagnose sampling problems
## 表示
fit
## Inference for Stan model: SevenScientist.
## 4 chains, each with iter=3000; warmup=1000; thin=1;
## post-warmup draws per chain=2000, total post-warmup draws=8000.
##
## mean se_mean sd 2.5% 25% 50% 75% 97.5% n_eff Rhat
## mu 9.88 0.01 0.12 9.60 9.81 9.86 9.95 10.06 128 1.04
## sig[1] 199.66 36.85 1979.60 16.77 28.48 46.86 92.32 854.54 2885 1.00
## sig[2] 51.23 14.52 788.07 2.85 5.52 9.37 14.24 144.60 2947 1.00
## sig[3] 63.59 53.92 1727.89 0.81 1.63 2.58 5.44 61.07 1027 1.00
## sig[4] 0.60 0.12 6.57 0.00 0.01 0.12 0.30 3.06 3119 1.00
## sig[5] 8.42 7.19 58.91 0.01 0.17 0.37 0.80 14.13 67 1.07
## sig[6] 0.56 0.14 5.37 0.00 0.04 0.13 0.34 2.28 1566 1.00
## sig[7] 2.45 1.27 16.51 0.00 0.11 0.29 0.62 10.07 170 1.02
## lp__ -3.55 0.58 3.10 -9.96 -5.55 -3.46 -1.36 1.83 29 1.14
##
## Samples were drawn using NUTS(diag_e) at Tue Mar 5 18:21:19 2019.
## For each parameter, n_eff is a crude measure of effective sample size,
## and Rhat is the potential scale reduction factor on split chains (at
## convergence, Rhat=1).
## 描画
plot(fit,pars=c("sig[1]","sig[2]","sig[3]","sig[4]",
"sig[5]","sig[6]","sig[7]"),show_density=T)
## ci_level: 0.8 (80% intervals)
## outer_level: 0.95 (95% intervals)
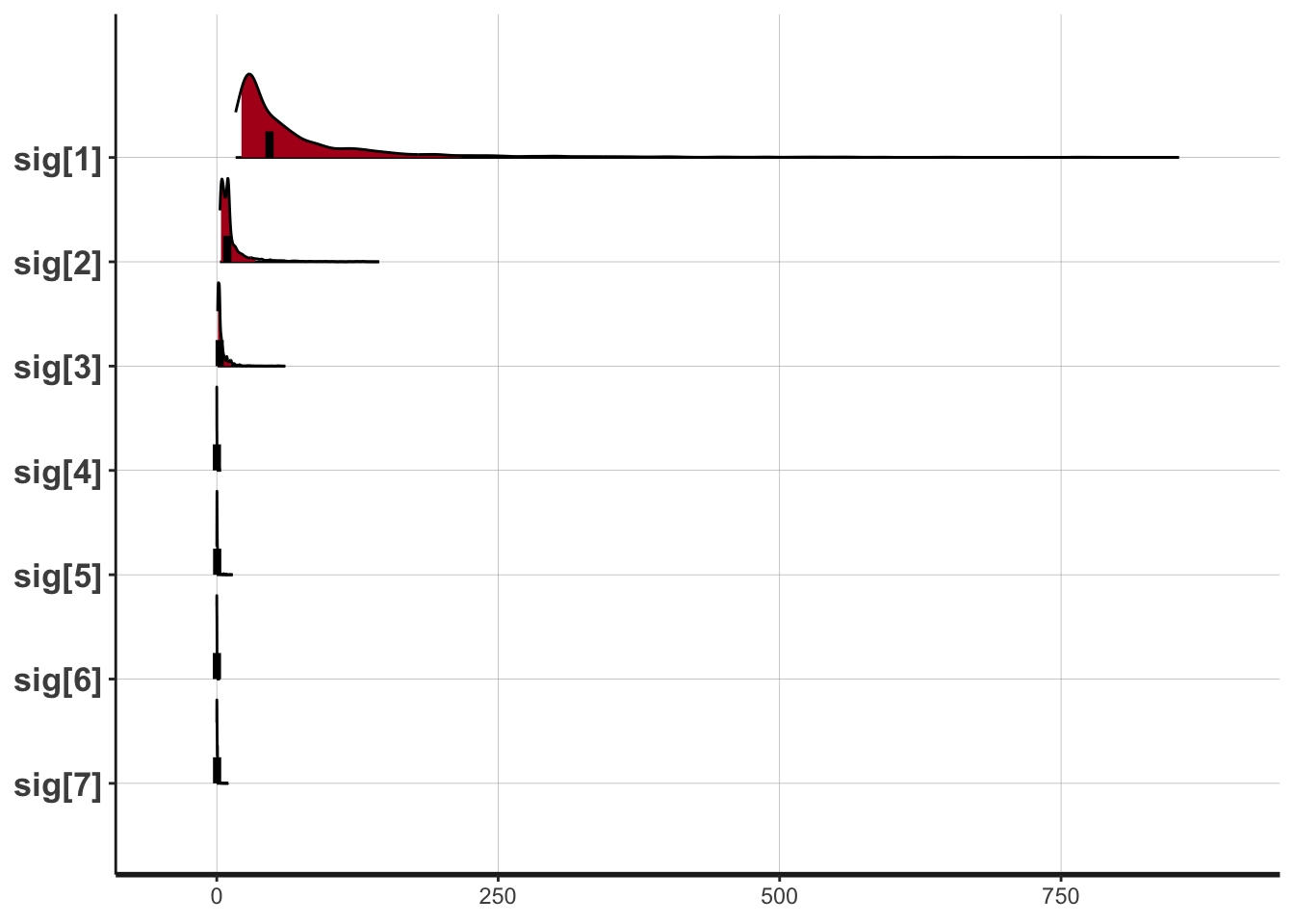